Beyond the Hype: Lessons on auditing AI systems from the front lines
Image from Shutterstock.
Two trends are dominating the world of AI: One is the rapid adoption of generative AI systems like ChatGPT, Bard and many others. The other hand is the growing legal requirements for AI audits, such as auditing mandates in New York City, which requires audits of AI systems used in the employment context, proposed laws at the state and federal levels in the US, as well as the EU AI Act.
At first glance, it can seem hard to reconcile these two trends. Generative AI systems are made up of billions or even trillions of parameters trained on vast amounts of data, and their complexity makes their outputs notoriously difficult to explain. But can complex generative systems undergo meaningful audits? The answer is yes. We have successfully audited a range of generative AI systems for bias. While auditing generative AI is not simple in practice, it is in fact possible—and even practical with the right mix of expertise, experience and expectations.
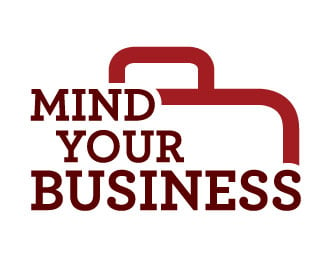
This article is based on our experience auditing AI systems as the first and only law firm specifically focused on AI risk management. Indeed, as a boutique law firm made up of both data scientists and lawyers, we have been applying our technical and legal expertise to AI systems for several years, and we have audited nearly every type of AI system, from traditional classifiers to graphs, generative AI models and others.
Here are five lessons we’ve learned.
1. Legal privilege is a critical asset
Let’s start with the role of lawyers in conducting AI audits (a subject we are very admittedly biased about). All too often, we see in-house lawyers take a back seat in technical matters. Lawyers may weigh in on activities to comply with various requirements but then hand over the responsibilities to more technical teams made up of data scientists or engineers. This is often a mistake.
Why are lawyers so important? Among the most overlooked reasons is legal privilege. Legal privilege allows companies engaged in sensitive matters to fully investigate and analyze potential risks without the fear of exposure over internal, exploratory discussions down the road. Companies need the protection to do diligent internal fact-finding to discover what has or could go wrong without fear that it can harm the company.
However, oversight of sensitive technical matters often gets delegated to nonlegal personnel, and privilege is unintentionally waived, meaning that information associated with the entire effort can be used against the company should litigation or external oversight occur. As is well established in cybersecurity, ensuring legal privilege is a critical aspect of any internal assessment of risks.
2. Legal standards exist for a reason; use them
Lawyers are central to AI audits for another reason: existing legal standards can and should be applied to managing AI risks. Regulations and case law around algorithmic bias have existed for over five decades in the areas of employment, housing and finance in the US. That precedent has created clear legal standards around bias that can be used and referred to—and companies that utilize this precedent can bolster their defensibility. In one of the few audits we have conducted that is publicly available, we applied these types of standards directly to a generative AI system with our colleagues at In-Q-Tel Labs (more information on that audit is available here).
More advanced and AI-specific research around AI bias management is active and developing rapidly—which is a welcome and needed development. However, many of these techniques are still in developmental stages, with no legal precedent or standing. This issue frequently arises during our AI audits, where data scientists apply cutting edge de-biasing techniques to AI that simply won’t stand up in the face of external legal oversight. In some cases, these techniques are just too new and too untested to be accepted by regulators.
3. Identify and collect data for testing
Another one of the most frequent issues we run into can feel like something of a catch-22: In the interest of good privacy practices, companies limit or avoid collection of sensitive data (such as race or ethnicity), but then realize that without it, they are less able to engage in adequate bias testing. It is not unusual for us to begin AI audits with data scientists and lawyers at a standstill over how to get the right data to test their AI. But companies still need to collect this type of data to adequately perform audits of their AI systems.
So what can companies do? They can resolve this issue in a host of ways. One of the most efficient ways is to infer it from the less-sensitive information companies do have on file. The most prominent method for this type of inference is known as Bayesian Improved Surname Geocoding, which has a long history in regulated areas such as consumer finance. BISG uses surnames and zip codes to infer protected information about gender and race or ethnicity. The Consumer Financial Protection Bureau has endorsed this approach—and endorsement from a major regulator helps establish legal defensibility should external scrutiny arise. There are alternatives that companies may explore as well, including a method that incorporates first names, known as BISFG, along with others.
Other ways to address missing demographic data include looking to data brokers to fill this gap which, as long as it aligns with applicable privacy policies, is another straightforward way to generate missing information. In some cases, our clients have reached out to select sets of customers or users, explained why they need this sensitive information, and simply asked for it directly.
It is important to note that while these recommendations and lessons have been established in application to traditional AI systems, they also apply to developing evaluations of generative AI systems as well. It just takes some creative thinking by lawyers and technologists working together to apply established standards to generative AI systems.
4. Who prepares the audit—and where does the audit go?
We’ve talked about the importance of lawyers, but just as important are those actually performing the audit. Sometimes this is driven by legal requirements, but depending on the circumstance, companies may want to perform audits internally or with external help. In other cases, the audit may have to be performed by external parties, which adds a level of nuance to the audit. What role and relationship should external auditors have, particularly if they must meet a particular legal definition for “independence”? Sometimes lawyers, such as outside counsel, can be the independent auditors. In other cases, that may be seen as a conflict of interest. Understanding the legal requirements that are driving the audit is a key factor in selecting who should actually undertake the auditing work.
Just as important is also understanding where the audit report is actually delivered. Will it be shared with third parties, such as business partners, vendors or regulators? Is it required to be available to the general public? These questions should be clarified before the audit begins. We typically divide our audit reports into two sections: The first details technical and legal analysis prepared for internal client review and use, which is typically covered by legal privilege; and a shorter, summary overview of the assessment intended for external dissemination.
5. What’s the point?
There are a wide variety of reasons companies perform AI audits. Some are directed toward compliance with evolving legal standards. Other audits demonstrate best-in-class efforts for the purposes of external oversight. Other audits take place to build trust with business partners and individual consumers. Understanding why the audit is taking place and how the information will be used are among the most important factors in ensuring an audit’s success.
While this might seem basic, it is surprising how easy this is to overlook. We’ve seen more than a few times, for example, audits recommend specific mitigation measures that don’t even make it back to the technical teams. Similarly, we’ve seen audits performed and made available for external dissemination that some company personnel thought were only for internal purposes.
With so much complexity—coordinating across teams, testing AI systems, acquiring the right data—it’s easy for communication issues to lead to problems down the road.
These five lessons are, of course, only a handful of what we’ve learned in doing risk assessments and auditing AI systems for bias. Auditing AI systems is a complex, nuanced task and can require some creativity from all involved, especially the legal and technical teams who are in the trenches to make it happen. For this reason, companies should be careful before and during an AI audit and make sure they seek out the right experts to ensure their audit accomplishes what they need it to.
Brenda Leong is a partner at BNH.AI. Andrew Burt is managing partner at BNH.AI.
Mind Your Business is a series of columns written by lawyers, legal professionals and others within the legal industry. The purpose of these columns is to offer practical guidance for attorneys on how to run their practices, provide information about the latest trends in legal technology and how it can help lawyers work more efficiently, and strategies for building a thriving business.
Interested in contributing a column? Send a query to [email protected].
This column reflects the opinions of the author and not necessarily the views of the ABA Journal—or the American Bar Association.